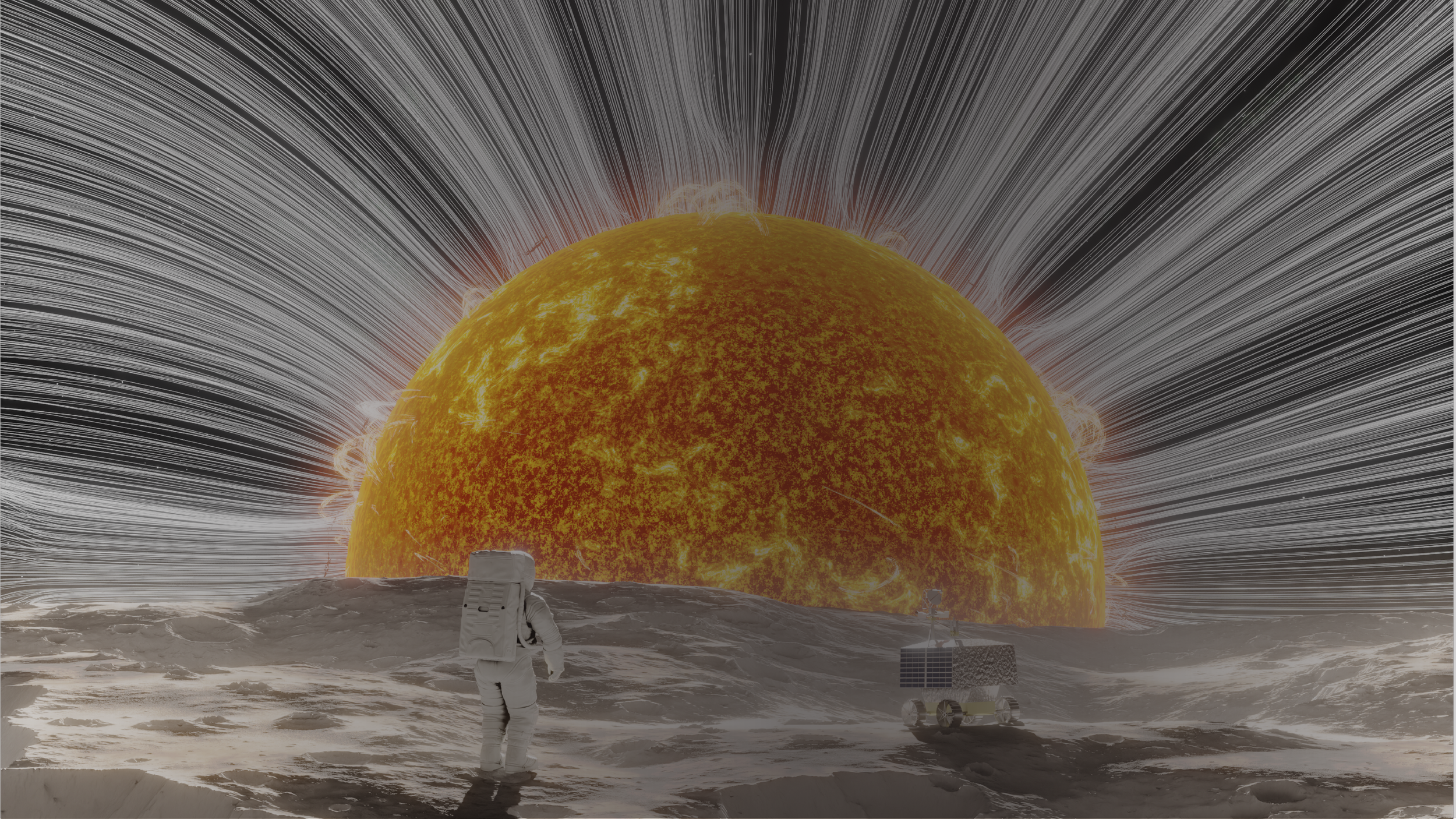
This work is supported through Grant #80NSSC24MO122 from SMD/NASA Heliophysics Division
RESEARCHER APPLICATIONS
are now open
New solar research shows the promise of AI for protecting lunar explorers and spaceship Earth
At NASA, artificial intelligence (AI) is in the process of revolutionizing space exploration and discovery, becoming a powerful new toolbox to deepen our understanding of the universe and better protect human activities in space.
Looking at our local star with fresh AIs.
Heliolab is a prime example of AI’s transformative utility for discovery and supporting NASA missions, a collaboration between NASA Google Cloud, NVIDIA, Pasteur Labs and administered by Trillium Technologies, focusing on breakthrough AI applications for heliophysics.
This highlights the critical need for AI for solar physics, as new methods play a key role in unraveling the complexities of Sun-Earth interactions. AI models are now essential in understanding how our Star’s behavior influences satellites, power grids, and communications on Earth, such as GPS. These models also assess how solar activity could affect missions like NASAs Artemis program heading to the Moon. As exploration extends to Mars and beyond, predicting the Sun’s behavior will become even more crucial. For example, can we forecast solar EUV irradiance at Mars when it is obscured behind the Sun from Earth’s view?
With AI the answer is yes.
“Space weather is an area of science where the rubber hits the road: something happens on the Sun, and it propagates 93 million miles to impact our planet”
- Madhulika Guhathakurta
NASA’s Living with a Star Program Scientist
A 3D Model of EUV for the inner solar system
This year one of the Heliolab team’s developed a groundbreaking 3D model of the Sun using data from the Solar Orbiter, Solar Dynamics Observatory (SDO), and STEREO A missions. Their advanced machine learning (ML) model, ITI (Instrument to Instrument Translation) integrates data from these satellites to create a coherent, multi-vantage view of the Sun. They also further developed the MEGS-AI neural network, which transforms this 3D model into an extreme ultraviolet solar irradiance forecast, allowing predictions to be made from any point in the solar system by placing virtual satellites in those locations.
This capability - providing Solar EUV irradiance predictions from any location in the solar - carries significant implications for space exploration. It enables scientists to forecast solar extreme ultraviolet (EUV) irradiance at Mars, helping NASA mission planners anticipate the environmental conditions that could impact spacecraft and instruments.
Predicting radiation dose for Artemis astronauts
NASA missions like the SDO monitor these events in real time, but until now, there hasn’t been a way to translate this data into actionable insight for astronaut safety. The SDOML pipeline, a dedicated analysis-ready ML data product, addresses this by generating predictions of space radiation conditions hours in advance. These early warnings can be sent to astronauts aboard the Lunar Gateway or on the lunar surface, allowing them to take protective measures such as shortening extravehicular activities (EVAs) or positioning the spacecraft between the Sun and themselves.
AI-powered foresight will be critical for safeguarding human crews working on or near the Moon and Mars, enhancing the safety of future space exploration missions.
AI is also emerging as a powerful tool for predicting solar energetic particles (SEPs), which pose a significant risk to astronauts. As NASA prepares for human missions to cis-lunar space and beyond, the ability to monitor and predict radiation levels in advance is crucial. Solar flares and coronal mass ejections (CMEs) release dangerous bursts of radiation that can expose astronauts to lifetime doses within minutes.
AI models are not only advancing radiation forecasting and solar EUV irradiance on Mars, but they are also showing promise for protecting Earth - particularly our technological civilization, which is vulnerable to solar flares and coronal mass ejections (CMEs), collectively known as solar eruptions. These events can cause vast magnetic fluctuations that can disrupt technological systems, posing a significant threat to Humanity often referred to as “the trillion dollar storm”.
Heliolab developed AI pipelines like DAGGER (Deep leArninG Geomagnetic pErtuRbation) and SHEATH to predict the effects of geomagnetic storms. DAGGER provides high-fidelity, real-time predictions of geomagnetic disturbances up to 30 minutes before their arrival at Earth, using solar wind observations from Lagrange Point 1 (L1). These predictions are vital for protecting satellites and communication systems from the disruptive impacts of solar storms. SHEATH complements DAGGER by offering longer-range predictions based on SDO data, providing a 5-7 day lead time on incoming solar wind conditions. Together, these models give both short-term alerts and longer-term forecasts.
To maintain model accuracy as new data emerges, Heliolab this year, developed GEOCLOAK, a continual learning system which improves the performance of DAGGER, SHEATH, and MEGS-AI. This adaptive system ensures that predictive AI tools stay updated and aligned with real-world conditions. This continual learning capability ensures the AI models remain effective through a range of distributions, future-proofing geoeffectiveness insights and enabling hyperlocal precision.
Protecting spaceship Earth
Improving Thermospheric Density Predictions in Low-Earth Orbit
AI is also crucial for managing the growing congestion in Earth’s orbit. Currently, around 13,000 active satellites are in orbit, with an additional 58,000 expected by 2030. Alongside these satellites, there are over 130 million pieces of space debris, creating a complex and hazardous environment. AI models are indispensable for predicting satellite movements and automating collision avoidance, helping to prevent potential collisions that could disrupt space operations.
As space becomes more crowded, AI-driven models will be essential for ensuring the safety and functionality of critical space services, including GPS, positioning, navigation and timing (PNT) systems, communications, Earth observation, space internet, scientific missions and human spaceflight.
Karman, an AI tool for measuring the fluctuations of the thermosphere, developed as part of Heliolab, is designed to create and benchmark data-driven models that outperform traditional empirical models in simulating thermospheric density. Currently, Karman is improving accuracy by 40-60% over state-of-the-art methods, providing a 100 minute advance warning. This demonstrates AI’s potential to enhance our understanding of rapidly changing space environments.
Traditional physical models, while highly accurate, are slow and computationally expensive, and often lack the necessary temporal resolution. AI, however, can process large volumes of observational data, uncovering patterns that numerical models might miss, AI-enhanced hybrid models, which integrate scientific insights with live data, are becoming a powerful tool for future space situational awareness.
According to NASA’s ML specialist Nat Mathews at the Goddard Space Flight Center, “Going forward, we're going to see more and more stuff like Karman, filling in the gaps where we have a ton of observational data from things like surface irradiance and in situ measurements, but an actual physical model at a realistic scale would be totally intractable.”
Karman is running live on SWxTREK here (100 mins in advance)
FDL-X Heliolab AI lead researcher Giacomo Accairini echoed this sentiment, “We were happily surprised when we started seeing that by adding new data, both space weather and solar, these models can perform better. It hints that there needs to be a lot of work in improving our models of Earth-Sun interaction. It also hints that these interactions are not well understood. There needs to be a lot more heliophysics research done since it’s still a poorly understood topic in terms of modeling.”
NASA’s growing use of AI marks a broader shift in the scientific community, where AI, initially met with skepticism, has proven invaluable in Heliophysics predictions. Hybrid models are now widely recognized as the future, critical for ensuring the safety of both crewed and uncrewed missions as space exploration expands further into the solar system. For example, Karman resolves the scenario that resulted in the loss of 38 Starlink satellites due to erroneous predictions of thermospheric drag.
With AI’s help, NASA’s understanding of solar-terrestrial interactions will continue to advance, providing crucial insights that enhance the safety of human missions across the solar system and protect our assets in Earth’s orbit from catastrophic solar events. AI’s role in space exploration is not just about downstream science; it is about ensuring a safer, smarter, and more efficient future. As NASA leads the way in AI integration, the future of space exploration will be defined by the synergy of cutting-edge technology and human ingenuity - for all Humankind.
Latest Publications
SuNeRF: 3D Reconstruction of the Solar EUV Corona Using Neural Radiance Fields
Abstract:
To understand its evolution and the effects of its eruptive events, the Sun is permanently monitored by multiple satellite missions. The optically thin emission of the solar plasma and the limited number of viewpoints make it challenging to reconstruct the geometry and structure of the solar atmosphere; however, this information is the missing link to understand the Sun as it is: a 3D evolving star. We present a method that enables a complete 3D representation of the uppermost solar layer (corona) observed in extreme ultraviolet (EUV) light. We use a deep-learning approach for 3D scene representation that accounts for radiative transfer to map the entire solar atmosphere from three simultaneous observations. We demonstrate that our approach provides unprecedented reconstructions of the solar poles and directly enables height estimates of coronal structures, solar filaments, coronal hole profiles, and coronal mass ejections. We validate the approach using model-generated synthetic EUV images, finding that our method accurately captures the 3D geometry of the Sun even from a limited number of 32 ecliptic viewpoints (∣latitude∣ ≤ 7°). We quantify the uncertainties of our model using an ensemble approach that allows us to estimate the model performance in the absence of a ground truth. Our method enables a novel view of our closest star and is a breakthrough technology for the efficient use of multi-instrument data sets, which paves the way for future cluster missions.
2024, American Astronomical Society
Improving Thermospheric Density Predictions in Low-Earth Orbit With Machine Learning
Abstract:
Thermospheric density is one of the main sources of uncertainty in the estimation of satellites' position and velocity in low-Earth orbit. This has negative consequences in several space domains, including space traffic management, collision avoidance, re-entry predictions, orbital lifetime analysis, and space object cataloging. In this paper, we investigate the prediction accuracy of empirical density models (e.g., NRLMSISE-00 and JB-08) against black-box machine learning (ML) models trained on precise orbit determination-derived thermospheric density data (from CHAMP, GOCE, GRACE, SWARM-A/B satellites). We show that by using the same inputs, the ML models we designed are capable of consistently improving the predictions with respect to state-of-the-art empirical models by reducing the mean absolute percentage error (MAPE) in the thermospheric density estimation from the range of 40%–60% to approximately 20%. As a result of this work, we introduce Karman: an open-source Python software package developed during this study. Karman provides functionalities to ingest and preprocess thermospheric density, solar irradiance, and geomagnetic input data for ML readiness. Additionally, it facilitates developing and training ML models on the aforementioned data and benchmarking their performance at different altitudes, geographic locations, times, and solar activity conditions. Through this contribution, we offer the scientific community a comprehensive tool for comparing and enhancing thermospheric density models using ML techniques.
2024, AGU
Physically Motivated Deep Learning to Superresolve and Cross Calibrate Solar Magnetograms
Abstract:
Superresolution (SR) aims to increase the resolution of images by recovering detail. Compared to standard interpolation, deep learning-based approaches learn features and their relationships to leverage prior knowledge of what low-resolution patterns look like in higher resolution. Deep neural networks can also perform image cross-calibration by learning the systematic properties of the target images. While SR for natural images aims to create perceptually convincing results, SR of scientific data requires careful quantitative evaluation. In this work, we demonstrate that deep learning can increase the resolution and calibrate solar imagers belonging to different instrumental generations. We convert solar magnetic field images taken by the Michelson Doppler Imager (resolution ∼2'' pixel−1; space based) and the Global Oscillation Network Group (resolution ∼2farcs5 pixel−1; ground based) to the characteristics of the Helioseismic and Magnetic Imager (resolution ∼0farcs5 pixel−1; space based). We also establish a set of performance measurements to benchmark deep-learning-based SR and calibration for scientific applications.
2024, American Astronomical Society
Heliophysics Publications
2024 - Grant #80NSSC24MO122 - SMD/NASA Heliophysics Division
Machine learning discovery of cost-efficient dry cooler designs for concentrated solar power plants - paper, Nature Journal
DOI 10.1038/s41598-024-67346-6SuNeRF: 3D Reconstruction of the Solar EUV Corona Using Neural Radiance Fields - Astrophysical Journal Letters (FDL USA 2022)
DOI 10.3847/2041-8213/ad12d2, Arxiv 2401.16388Improving Thermospheric Density Predictions in Low-Earth Orbit With Machine Learning- AGU Space Weather Volume 22, Issue 2
DOI 10.1029/2023SW003652Physically Motivated Deep Learning to Superresolve and Cross Calibrate Solar Magnetograms - The Astrophysical Journal
DOI 10.3847/1538-4365/ad12c2Virtual EVE: a Deep Learning Model for Solar Irradiance Prediction - Paper
Arxiv 2408.17430v1Multiscale Geoeffectiveness Forecasting using SHEATH and DAGGER - Poster, Astronomical Society of India 2024
2023
A Scientific Cloud Computing Platform for Ingestion and Processing of SDO Data - 4th Eddy Cross-Disciplinary Symposium (FDL-X 2023)
AIA is All You Need: SDO MEGS A&B virtualization via Convolutional Deep Learning - 4th Eddy Cross-Disciplinary Symposium (FDL-X 2023)
A Novel Synthesis of SDO EVE MEGS A&B Spectral Irradiance Data through Convolutional Deep Learning - AGU 2023 (FDL-X 2023)
A Scientific Cloud Computing Platform for Ingestion and Processing of SDO Data - DASH 2023 (FDL-X 2023)
Virtual EVE: a Deep Learning Model for Solar Irradiance Prediction - NeurIPS 2023 (FDL-X 2023)
AI Inference Products, Foundation Models and multi-domain approaches to NASA Heliophysics - 4th Eddy Cross-Disciplinary Symposium (FDL-X Helio)
Multiscale Geoeffectiveness Forecasting using SHEATH and DAGGER - 4th Eddy Cross-Disciplinary Symposium (FDL-X 2023)
Multiscale Geoeffectiveness Forecasting: Upgrading the DAGGER Pipeline - AGU 2023 (FDL-X 2023)
Improving thermospheric drag modeling with EUV images: an FDL-X 2023 project - 4th Eddy Cross-Disciplinary Symposium (FDL-X 2023)
Incorporating Direct EUV Irradiance from Solar Images into Thermospheric Density Modelling with Machine Learning - AGU 2023 (FDL-X 2023)
High-Cadence Thermospheric Density Estimation enabled by Machine Learning on Solar Imagery - NeurIPS 2023 (FDL-X 2023)
Karman - a Machine Learning Software Package for Benchmarking Thermospheric Density Models - AMOS 2023 (FDL-X 2023)
A Federated Distributed Learning Benchmark for Solar Wind Speed Forecasting Using Solar EUV Images - Space Weather Workshop 2023 (FDLUSA 2022)
2022
Global Geomagnetic Perturbation Forecasting Using Deep Learning - AGU Space Weather Volume 20, Issue 6
DOI 10.1029/2022SW003045, Arxiv 2205.12734v1
2021
Modeling and forecasting ground geomagnetic perturbations using deep learning on spherical harmonics - COSPAR2021 Machine Learning for Space Sciences (You Tube Link) (FDL USA 2020)
Multichannel autocalibration for the Atmospheric Imaging Assembly using machine learning - Astronomy & Astrophysics Journal (FDL USA 2019)
DOI 10.1051/0004-6361/202040051, Arxiv 2012.14023v4Auto-Calibration and High-Fidelity Virtual Observations of Remote Sensing Solar Telescopes with Deep Learning - JPL AI and Data Science Workshop 2021 (FDL USA 2019) [I]
Correlation of Auroral Dynamics and GNSS Scintillation with an Autoencoder - JPL AI and Data Science Workshop 2021 (FDL USA 2019) [I]
Autonomous deep-space missions: can deep learning be used to optimize data transmission - COSPAR 2021 (FDL USA 2019)
Automating the Calibration of the Atmospheric Imaging Assembly - COSPAR 2021 (FDL USA 2019)
Forecasting Ground Magnetic Perturbation Using Deep Learning on Spherical Harmonics - American Meteorological Society 101th annual meeting 2021 (FDL USA 2020)
2020
Multi-Channel Auto-Calibration for the Atmospheric Imaging Assembly using Machine Learning- Paper at AGU 2020 (FDL USA 2019)
Determining new representations of “Geoeffectiveness” using deep learning - AGU 2020 (FDL USA 2020)
Multi-Channel Auto-Calibration for the Atmospheric Imaging Assembly instrument with Deep Learning- AGU 2020 (FDL USA 2019)
RotNet: Fast and Scalable Estimation of StellarRotation Periods Using Convolutional NeuralNetworks- NeurIPS 2020 ML4PS (Physical Sciences) Workshop (FDL USA 2020)
Global Earth Magnetic Field Modeling and Forecasting with Spherical Harmonics Decomposition- NeurIPS 2020 ML4PS (Physical Sciences) Workshop (FDL USA 2020)
2019
Using U-Nets to create high-fidelity virtual observations of the solar corona - NeurIPS Workshop 2019 (FDL USA 2019)
Arxiv 1911.04006v1A deep-learning based approach for predicting high latitude ionospheric scintillations using geospace data and auroral imagery - JPL NASA Abstract (FDL USA 2019)
Auto-Calibration of Remote Sensing Solar Telescopes with Deep Learning- NeurIPS Workshop 2019 (FDL USA 2019)
Arxiv 1911.04008v1Single-Frame Super-Resolution of Solar Magnetograms: Investigating Physics-Based Metrics & Losses- NeurIPS Workshop 2019 (FDL USA 2019)
Arxiv 1911.01490v1Correlation of Auroral Dynamics and GNSS Scintillation with an Autoencoder- NeurIPS Workshop 2019 (FDL USA 2019)
Arxiv 1910.03085v1Prediction of GNSS Phase Scintillations: A Machine Learning Approach - NeurIPS Workshop 2019 (FDL USA 2019)
Arxiv 1910.01570v1A deep learning Approach to forecast Tomorrow's Solar Wind Parameters- AGU 2019 (FDL USA 2019)
Enhancing the Predictability of GNSS Scintillations - AGU 2019 (FDL USA 2019) [I]
Auto-calibration and reconstruction of SDO’s Atmospheric Imaging Assembly channels with Deep Learning- AGU 2019 (FDL USA 2019)
A deep learning virtual instrument for monitoring extreme UV solar spectral irradiance - Science Advances 2019 (FDL USA 2018)
DOI 10.1126/sciadv.aaw6548A machine learning dataset prepared for NASA’s Solar Dynamics Observatory- Astrophysical Journal 2019 (FDL USA 2018)
DOI 10.3847/1538-4365/ab1005, Arxiv 1903.04538v1